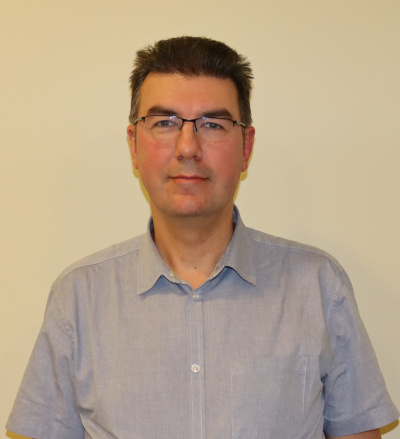
‘This year's Nobel Prize winners Baker, Hassabis and Jumper used an approach that is de facto an extension of three previously known methods: homology modelling, sequence threading and dynamic fragment assembly. In this situation, having at their disposal quite a large database of sequences, they applied machine learning methods to approximate an unknown structure based on already known ones,' comments the Vice-Dean for Development and Informatisation of the Faculty of Chemistry, dr hab. Artur Giełdoń, prof. UG.
As you can read on the Nobel Committee website, the 2024 prize was awarded to David Baker ‘for computational protein design’ and Demis Hassabis and John Jumper ‘for protein structure prediction’. In other words, David Baker received the prize for computational protein design, while Demis Hassabis and John Jumper received the prize for protein structure prediction.
When we talk about proteins, most of us think of their nutritional properties. ‘Eat yoghurt - it's protein; eat cooked meat - it's protein; don't eat this, it's sugar or fat itself’. Our bodies are built in such a way that a balance in the consumption of all the elements listed (and many not listed) must be maintained for them to function properly. This is where most of us' knowledge ends. However, underneath the whole mantle of dietetics, all living organisms are complex biochemical machines whose functioning we are still trying to understand.
Let's focus on proteins - the subject of this year's Nobel Prize. In the simplest terms, we can think of them as small (size 2-100 nm (1nm=10-9m)), highly specialised nanorobots. Some will build our muscles and be responsible for their movement, others will repair damaged tissues, and others will be responsible for digesting food and even for the process of seeing and perceiving our surroundings. Thus, without proteins, no living organism can exist. Even viruses, which can hardly be called ‘alive’, are also made up of proteins. This is why learning about them is so important.
For our purposes, let us imagine a protein as an excavator that is supposed to dig the foundation for a house. The operator gets into the machine, puts the key in the ignition and does his job. And this is what happens in the normal case. Let us now imagine two extreme cases. The first is that someone has taken the key; the second is that 20 excavators have been delivered to the construction site instead of one. What do we need to do? In the first case, provide the key - so that the machine can be activated, in the second case, put a stick in the ignition - because the excess excavators will dig too big a hole. This is how most drugs work: it shifts the balance between the inactive and active forms of proteins. This begs the question of how to design the right key (drug).
From the early days of biochemistry, this was done in such a way that a plant was known to alleviate the effects of a disease. Its constituents were isolated and tested empirically on masses of volunteers (volunteer mice, willing to give their lives in the service of biochemistry). Today, we know much more. We already know which proteins are responsible for processes in our bodies. Unfortunately, as is so often the case in life, this is where we hit a technical hurdle. While the sequencing of genes responsible for coding proteins is now almost a routine process, the process of obtaining the spatial structure of proteins is very complicated. We only need to compare the number of database entries - UniProt (sequence) 254 million; PDB (spatial structure) 230 thousand - to imagine how little we still know and how many discoveries await us. At this point, it must be added that a protein with 200 amino acid residues may have 20,200 possible sequence combinations. And this is where the role of this year's Nobel laureates begins. As an aside, it should be added that this is a continuation of the prize awarded in 2013 to Martin Karplus, Michael Levit and Arieh Warshel, which also concerned the process of obtaining the spatial structure of proteins. In my personal opinion, the big absentee from the 2013 Nobel Prize was the late Harold Scheraga.
Returning to the subject, the problem of the huge discrepancy between the number of spatial structures and the number of sequences was seriously noticed in 1994, when the first experiment (the organisers didn't like the word competition, but what's an experiment where there is a prediction ranking) on the theoretical prediction of spatial structures of proteins based only on sequences was announced (website predictioncenter.org). One of the people who actively organise it is a Pole - Krzysztof Fidelis. The experiment involves experimental groups sending the obtained spatial structures of proteins in secret. On the CASP website, however, it is possible to download a protein sequence and then send a response in the form of a three-dimensional model. The organisers compare this model with the structure they have and rank the predictions based on this. The Gdańsk group, of which I am a member, has participated in this experiment many times with considerable success. Thus, I dare say that it was this initiative that stimulated the scientific community and drove progress in the theoretical prediction of spatial structures of proteins.
The work of Baker, Hassabis and Jumper involves harnessing machine learning methods to derive spatial structures of proteins. Two strands of research need to be distinguished here. Baker won a prize for methods that enable protein design (Nature, 2016, vol. 537, 320-327) (Baker also did very well with his Rosetta programme). Hassabis and Jumper for their method for obtaining spatial structures of proteins (Nature, 2021, vol. 596, 583-589). At the moment, the situation is such that even with a home computer (the desktop at which I work has a 10-core processor, RTX 4090 and 96 GB of memory), anyone can predict the spatial structure of the protein under study on their own. Such a computer does it in 3-6 hours, depending on the length of the sequence. And this is progress on the scale of a Nobel Prize.
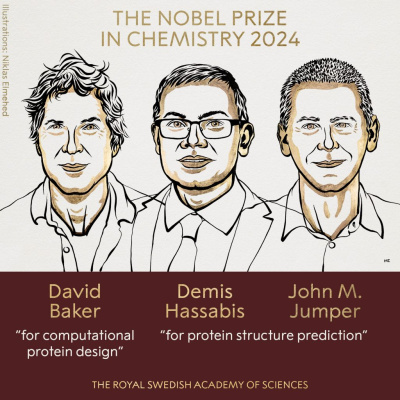
il. Niclas Emelhed
The slightly duller science part
In a basic biochemistry course, students are taught about Anfinsen's thermodynamic hypothesis, which states that the lowest-energy protein structure adopts the native conformation. In computational programmes, and due to the huge number of atoms we are mainly talking about empirical force fields, we have a well-defined energy function. Among other things, it depends on the values of bond lengths, valence and torsion angles, as well as electrostatic and van der Waals interactions. So, if we can determine the energy of a protein well, what is the problem? Well, there are two problems. For our purposes, we can imagine the graph of such a function, which we will call the potential energy hypersurface graph, as a golf course with mountains, valleys and holes. And this is the kind of image that our human minds are capable of ‘grasping’ - a three-dimensional space. The great Polish mathematician Stefan Banach saw five dimensions and that was the end of it. Here the problem is that our function will have 3n-6 (where n will be equal to the number of atoms) degrees of freedom. This means that this is how many dimensions the energy function whose minimum we want to find will actually have. To keep things simple, the minimum of the potential energy rarely corresponds to the native structure of the protein. In his lectures, prof. Harold Scheraga used to draw a diagram with two very well-defined minima. The first was broad and not very deep, the second sharp and very deep. So, where do we find the native structure we are looking for? In the more likely place - that is, wide and not too deep. That is, in the place with the lowest free energy, not potential energy. Let's imagine a running dog on our golf course and the fact that a bone has been buried near the last hole. The dog will run around the hole sniffing fiercely (we can call this chemical potential), itself being at a minimum (assuming native structure). However, as it happens in life, our golf course was built on a site where there used to be a farm with its own well. The well was protected, but over the years the boards had decayed, and as a consequence, our dog fell in there. The dog would be stuck in the well until someone rescued it. This is, in simple terms, the difference between potential energy and free energy. It is the same with proteins. The organism defends itself against improperly folded proteins by repairing them with chaperone proteins or by destroying them, as improperly folded proteins can do more harm than good. The method described above for obtaining spatial structures of proteins is applied to a whole host of different types of force fields. One of these is even being developed in the Gdansk Group, under the direction of prof. Adam Liwo. This is the UNRES force field.
This year's Nobel laureates - Baker, Hassabis and Jumper - used a different approach, which is de facto an extension of three previously known methods: homology modelling, threading and dynamic fragment assembly. In this situation, with an already quite large database of sequences at their disposal, they applied machine learning methods to approximate an unknown structure based on already known ones. The database of spatial structures of proteins already held is large enough that the process was successful. In contrast to the method described earlier, in this case, huge computing power and a supercomputer are not needed. Instead, what is needed is an SSD of at least 4TB and a good graphics card.
On the other hand, looking through the UniProt database (in which a great many computer-derived models are deposited), we can see that some of them have unstructured fragments (this is visible in the last column of the PDB file, where the probability of guessing a given structural fragment is given). So there is still more to work on.
Just as the big absentee in 2013 was Harold Scheraga, the big absentee in 2024 is Yang Zhang, whose group developed the I-Tasser programme. The paths of the Nobel Committee are unknown....