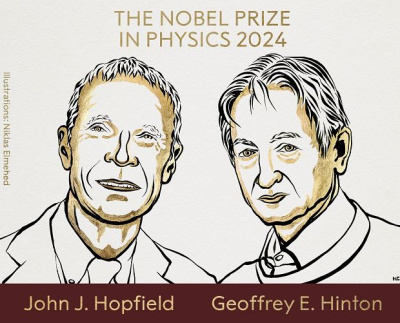
The 2024 Nobel Prize in Physics was not awarded for research that makes fundamental contributions to our understanding of the world, nor was it awarded for groundbreaking experimental methods. Dr Paweł Mazurek, Deputy Director of the Institute of Informatics at WMFI UG, tells us what guided the Nobel Committee this year.
The Nobel Committee awarded the 2024 Nobel Prize in Physics to John J. Hopfield and Geoffrey E. Hinton ‘for their fundamental discoveries and inventions enabling machine learning using artificial neural networks’. This is a unique award.
For one thing, the award-winning achievements do not fall into the category of those that are per se fundamental contributions to our understanding of the world: unlike such honours of recent years as those for the ‘discovery of a supermassive compact object at the centre of our galaxy’ (2020), ‘theoretical discoveries in physical cosmology’ (2019), or ‘theoretical discoveries in the field of topological phase transitions and topological phases of matter’ (2016).
Nor did the Nobel College go down the route of singling out experimental methods that are associated with such fundamental insights: ‘for experiments with entangled photons, establishing violations of Bell's inequality and pioneering quantum computing’ (2022), “for experimental methods generating attosecond pulses of light to study electron dynamics in matter’ (2023), or “for optical tweezers and their application to biological systems’ (2018).
Mean physicists may even point out that this year's prize is reminiscent of the one in 1912, awarded ‘for the invention of automatic regulators to be used in conjunction with gas tanks to power lighthouses and light buoys’ - an achievement that was important from an industrial point of view at the time, but which did not represent a breakthrough in physics, nor one that managed to lead to such a breakthrough before the prize was awarded.
So what guided the Committee in its choice this year?
As highlighted in the explanatory memorandum, John J. Hopfield drew inspiration from models of ferromagnetic materials when he proposed his dynamic model of associative memory in 1982 (he now receives the prize at the age of 91) - and the associative memory model itself was a fundamental contribution to our knowledge of the computational capabilities of neural networks. Geoffrey E. Hinton, by developing a stochastic generalisation of the Hopfield model, called a Bolztmann machine, and in particular, by proposing efficient algorithms for learning neural networks in Bolztmann machines with ties (2002), laid the foundations for the development of deep machine learning methods.
In addition to the physical inspiration behind artificial neural network models, the Committee emphasises their application in other scientific fields. In particular, the use of artificial neural networks makes it possible to simulate physical systems with the accuracy to predict the structure and dynamics of new materials or to increase the accuracy of climate models without excessive use of computing power to perform calculations. Deep neural networks are also being used to predict the three-dimensional structures of proteins.
At the same time, attention is drawn to the often overlooked in public perception and growing role that artificial neural networks play in the analysis of experimental data - networks were used in analyses enabling the detection of the Higgs boson in 2012 or the creation of a neutrino map of the Milky Way based on data collected by the IceCube neutrino detector at the South Pole.
In this sense, this year's award can be seen as part of a trend of recognising the provision of tools to further our knowledge of the world, where the importance of data analysis tools is already on a par with the importance of experimental techniques, which we have become accustomed to rewarding.
The pervasive presence of artificial neural networks, whether for image recognition, speech processing or numerous applications in medical technology, allows us to hope that the social impact of the award-winning methods will also be incomparably greater than that of ‘automatic regulators to be used in conjunction with gas tanks to power lighthouses and light buoys’.